Lisa Wimmer
About
I started as a PhD student at the Statistical Learning & Data Science working group in February 2022.
I obtained a Bachelor's degree (B.A.) in Business Administration from DHBW Ravensburg and a Bachelor's and consecutive Master's degree (B.Sc., M.Sc.) in Statistics from LMU Munich.
My main research focus is on the quantification of uncertainty, where I am active in our research subgroups Probabilistic Machine and Deep Learning and Causal and Fair Machine Learning.
Furthermore, I am part of the Data Science Group chaired by Prof. David Rügamer.
I receive funding from the Konrad Zuse School of Excellence in Reliable AI (relAI).
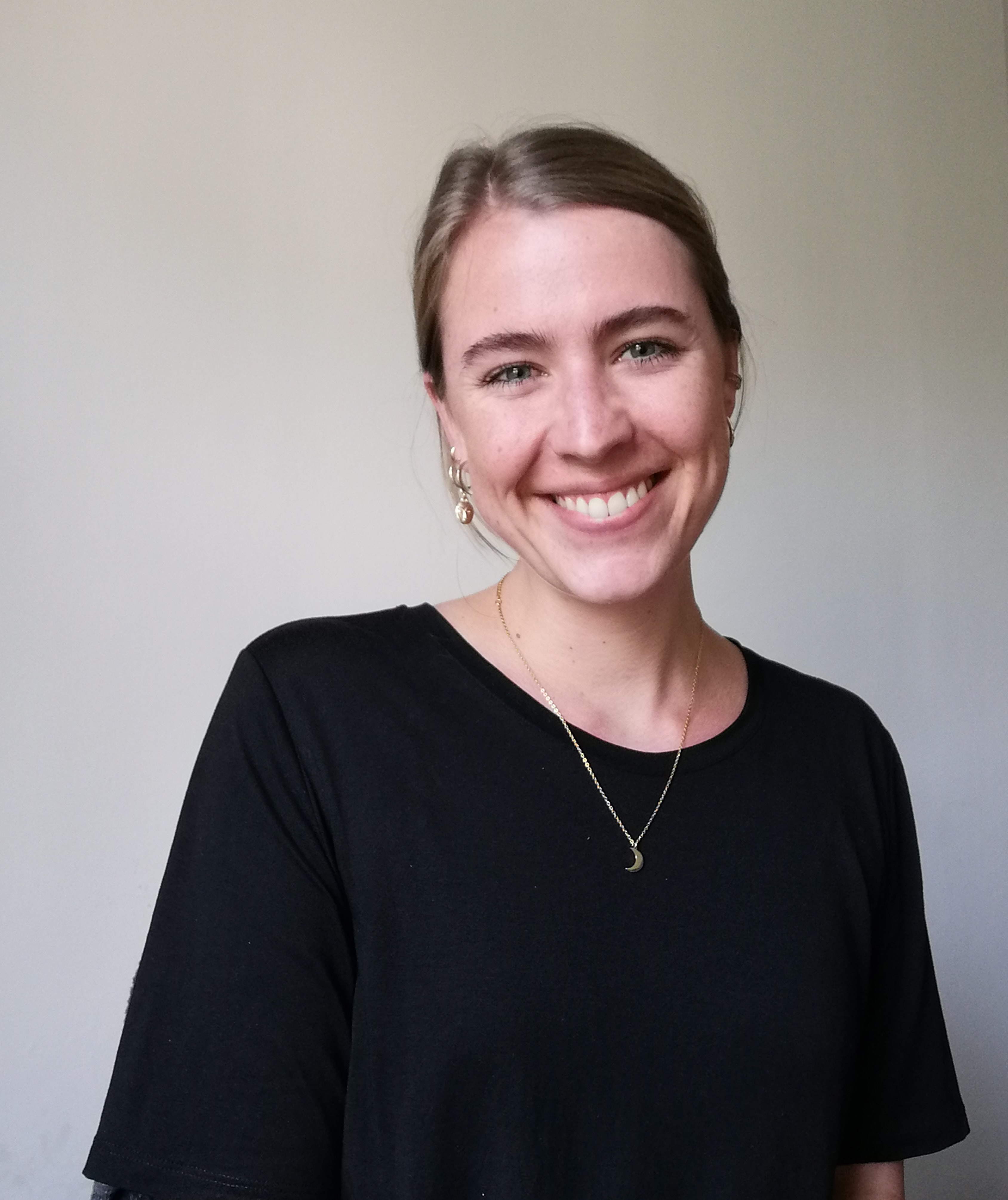
Contact
Institut für Statistik
Ludwig-Maximilians-Universität München
Ludwigstraße 33
D-80539 München
lisa.wimmer [at] stat.uni-muenchen.de
Research interests
- Predictive uncertainty
- Probabilistic machine learning
- Bayesian deep learning
- Uncertainty and causal inference
- Imprecise probabilities
- Weak supervision
You can find me on
References
- Vahidi A, Wimmer L, Gündüz HA, Bischl B, Hüllermeier E, Rezaei M (2024) Diversified Ensemble of Independent Sub-Networks for Robust Self-Supervised Representation Learning Machine Learning and Knowledge Discovery in Databases (ECML-PKDD), Springer International Publishing.
link|pdf. - Sale Y, Hofman P, Löhr T, Wimmer L, Nagler T, Hüllermeier E (2024) Label-wise Aleatoric and Epistemic Uncertainty Quantification 40th Conference on Uncertainty in Artificial Intelligence (UAI),
link|pdf. - Sommer E, Wimmer L, Papamarkou T, Bothmann L, Bischl B, Rügamer D (2024) Connecting the Dots: Is Mode-Connectedness the Key to Feasible Sample-Based Inference in Bayesian Neural Networks? 41st International Conference on Machine Learning (ICML),
link|pdf. - Wiese JG, Wimmer L, Papamarkou T, Bischl B, Günnemann S, Rügamer D (2024) Towards Efficient MCMC Sampling in Bayesian Neural Networks by Exploiting Symmetry (Extended Abstract) 33rd International Joint Conferences on Artificial Intelligence (IJCAI),
link|pdf. - Vahidi A, Schosser S, Wimmer L, Li Y, Bischl B, Hüllermeier E, Rezaei M (2024) ProSMIN: Probabilistic Self-supervised Representation Learning via Scoring Rules Minimization 12th International Conference on Learning Representations (ICLR),
link|pdf. - Wiese JG, Wimmer L, Papamarkou T, Bischl B, Günnemann S, Rügamer D (2023) Towards Efficient MCMC Sampling in Bayesian Neural Networks by Exploiting Symmetry Machine Learning and Knowledge Discovery in Databases (ECML-PKDD), Springer International Publishing.
link|pdf. - Bothmann L, Wimmer L, Charrakh O, Weber T, Edelhoff H, Peters W, Nguyen H, Benjamin C, Menzel A (2023) Automated wildlife image classification: An active learning tool for ecological applications. Ecological Informatics 77.
link|pdf. - Wimmer L, Sale Y, Hofman P, Bischl B, Hüllermeier E (2023) Quantifying Aleatoric and Epistemic Uncertainty in Machine Learning: Are Conditional Entropy and Mutual Information Appropriate Measures? 39th Conference on Uncertainty in Artificial Intelligence (UAI),
link|pdf.