Hüseyin Anil Gündüz
About
I am a Ph.D. student at the Chair of Statistical Learning and Data Science at LMU Munich since June 2020.
I received my BSc degrees in both Electrical Engineering and Physics from Bogazici University, Istanbul.
I completed an MSc degree in Communications Engineering at TU Munich.
My doctoral research is on the GenomeNet project, in collaboration with the Computational Biology of Infection Research team in the Helmholtz Centre for Infection Research. The project aims to develop suitable deep learning models for sequential genomics data. So far, I have worked on self-supervised learning, uncertainty quantification, and neural architecture search. Self-supervised learning techniques utilize big unlabeled datasets to boost the performance of machine learning models, especially when the number of labeled data samples is limited [1,4,8]. Uncertainty quantification in machine learning targets evaluation and reduction of uncertainties in decisions of machine learning models [1,2,7]. Neural architecture search discovers powerful neural architectures automatically [3,6].
I am part of the Methods Beyond Supervised Learning and Probabilistic Machine and Deep Learning research groups.
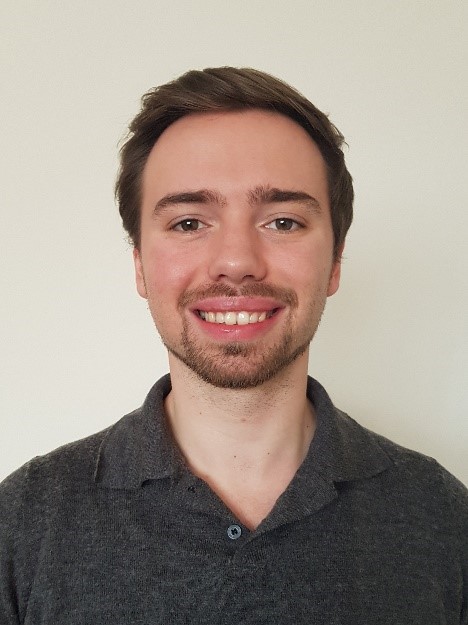
Contact
Institut für Statistik
Ludwig-Maximilians-Universität München
Ludwigstraße 33, D-80539 München
anil.guenduez [at] stat.uni-muenchen.de
Research Interests
- Deep Learning for Genomics Data
- Self-supervised Learning
- Uncertainty Quantification
- Neural Architecture Search
- Time Series Problems
You Can Find me on
References
- Vahidi, A., Wimmer, L., Gündüz, H. A., Bischl, B., Hüllermeier, E., Rezaei, M. (2023). Diversified Ensemble of Independent Sub-Networks for Robust Self-Supervised Representation Learning.
pdf - Gündüz, H. A., Giri, S., Binder, M., Bischl, B., & Rezaei, M. (2023). Uncertainty Quantification of Deep Learning Models for Predicting the Regulatory Activity of DNA Sequences. IEEE International Conference on Machine Learning and Applications (ICMLA 2023).
- Scheppach, A., Gündüz, H. A., Dorigatti, E., Münch, P. C., McHardy, A. C., Bischl, B., Rezaei, M., Binder, M. (2023). Neural Architecture Search for Genomic Sequence Data. IEEE Conference on Computational Intelligence in Bioinformatics and Computational Biology (CIBCB 2023).
link- Gündüz, H. A., Binder, M., To, X.-Y., Mreches, R., Bischl, B., McHardy, A. C., Münch, P. C. & Rezaei, M. (2023). A self-supervised deep learning method for data-efficient training in genomics. Nature Portfolio - Communications Biology 6, 928 (2023).
link | pdf- Mreches, R., To, X.-Y., Gündüz, H. A., Moosbauer, J., Klawitter, S., Deng, Z.-L., Robertson, G., Rezaei, M., Asgari, E., Franzosa, E. A., Huttenhower, C., Bischl, B., McHardy A. C., Binder, M., Münch, P. C. (2023). A platform for deep learning on (meta)genomic sequences.
link | pdf - Scheppach, A., Gündüz, H. A., Dorigatti, E., Münch, P. C., McHardy, A. C., Bischl, B., Rezaei, M., Binder, M. (2023). Neural Architecture Search for Genomic Sequence Data. IEEE Conference on Computational Intelligence in Bioinformatics and Computational Biology (CIBCB 2023).
- Gündüz, H. A., Mreches, R., Moosbauer, J., Robertson, G.,
To, X.-Y., Franzosa, E. A., Huttenhower, C., Rezaei, M., McHardy, A. C. Bischl, B. Münch, P. C., Binder, M. (2023) Optimized model architectures for deep learning on genomic data.
link | pdf - Turkoglu, M. O., Becker, A., Gündüz, H. A., Rezaei, M., Bischl, B., Daudt, R. C., D’Aronco, S., Wegner, J. D., & Schindler, K. (2022). FiLM-Ensemble: Probabilistic Deep Learning via Feature-wise Linear Modulation. Advances in Neural Information Processing Systems (NeurIPS 2022).
link | pdf - Hurmer, N., To, X.-Y., Binder, M., Gündüz, H. A., Münch, P. C., Mreches, R., McHardy, A. C., Bischl, B., & Rezaei, M. (2022). Transformer Model for Genome Sequence Analysis. LMRL Workshop - NeurIPS 2022.
link | pdf