Emilio Dorigatti
About
I have a Bachelor's in Computer Science and a double Master's degree (from the Technical University of Eindhoven and from the Royal Institute of Technology, Stockholm) in Data Science obtained through the EIT Digital Master's School, as well as a minor degree in Innovation & Entrepreneurship.
My PhD is about incorporating uncertainty in the design of personalized vaccines for cancer, done in collaboration with the Institute of Computational Biology at the Helmholtz Zentrum. It revolves around two central topics: (1) design vaccines through discrete optimization, in particular mixed integer linear (stochastic) programming, and (2) improve the predictions of pivotal quantities, with a particular focus on uncertainty, needed to design vaccines, mainly through deep semi-supervised and/or multiple-instance learning.
I am part of the Methods Beyond Supervised Learning and Probabilistic Machine and Deep Learning research groups, as well as the Translational Immunoinformatics research group at the Institute of Computational Biology, Helmholtz Zentrum.
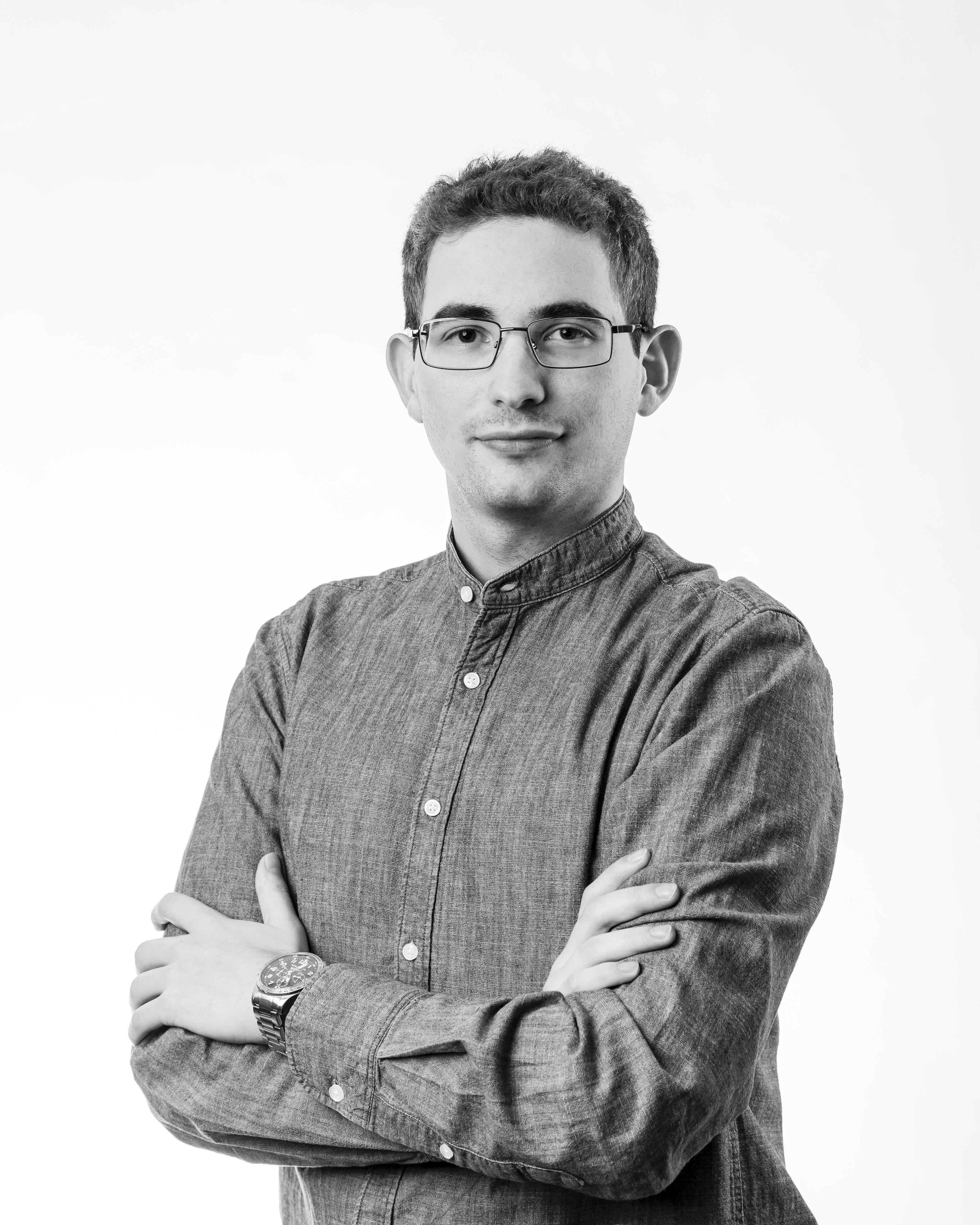
Research Interests
- Uncertainty Quantification
- Bayesian Deep Learning
- Discrete Optimization
- Linear Programming
- Positive-unlabeled learning
- Multiple-instance learning
Teaching
- Applied Deep Learning with TensorFlow and Pytorch SS22
- Deep Learning WS21/22
- Uncertainty Quantification in Deep Learning WS20/21
- Deep Learning WS20/21
- Deep Learning WS19/20
Awards
- Best poster at RECOMB2020
You Can Find me on
Publications
- Dorigatti E, Bischl B, Rügamer D (2023) Frequentist Uncertainty Quantification in Semi-Structured Neural Networks International Conference on Artificial Intelligence and Statistics, PMLR.
- Dorigatti E, Bischl B, Schubert B (2022) Improved proteasomal cleavage prediction with positive-unlabeled learning. Extended Abstract presented at Machine Learning for Health (ML4H) symposium 2022, November 28th, 2022, New Orleans, United States & Virtual abs/2209.07527.
link | pdf. - Ziegler I, Ma B, Nie E, Bischl B, Rügamer D, Schubert B, Dorigatti E (2022) What cleaves? Is proteasomal cleavage prediction reaching a ceiling? Extended Abstract presented at the NeurIPS Learning Meaningful Representations of Life (LMRL) workshop 2022.
link | pdf. - Dorigatti E, Goschenhofer J, Schubert B, Rezaei M, Bischl B (2021) Positive-Unlabeled Learning with Uncertainty-aware Pseudo-label Selection. arXiv preprint arXiv:2201.13192.
link|pdf. - Rezaei M, Dorigatti E, Rugamer D, Bernd Bischl and (2022) Joint Debiased Representation Learning and Imbalanced Data Clustering IEEE International Conference on Data Mining (ICDM) Deep Learning and Clustering (DLC) Workshop,
- Boniolo F, Dorigatti E, Ohnmacht AJ, Saur D, Schubert B, Menden MP (2021) Artificial intelligence in early drug discovery enabling precision medicine. Expert Opinion on Drug Discovery, 1–17.
link | pdf. - Fritz C, Dorigatti E, Rügamer D (2021) Combining Graph Neural Networks and Spatio-temporal Disease Models to Predict COVID-19 Cases in Germany. arXiv:2101.00661 [cs, stat].
link|pdf. - Dorigatti E, Schubert B (2020) Graph-theoretical formulation of the generalized epitope-based vaccine design problem (RD Kouyos, Ed.). PLOS Computational Biology 16, e1008237.
link | pdf. - Dorigatti E, Schubert B (2020) Joint epitope selection and spacer design for string-of-beads vaccines. Bioinformatics 36, i643–i650.
link | pdf. - Kahn J, Dorigatti E, Lieret K, Lindner A, Kuhr T (2020) Selective background Monte Carlo simulation at Belle II. EPJ Web Conf. 245, 02028.
link | pdf. - Fossati M, Dorigatti E, Giuliano C (2018) N-ary relation extraction for simultaneous T-Box and A-Box knowledge base augmentation (PE Cimiano, Ed.). Semantic Web 9, 413–439.
link | pdf.
Contact
Institut für Statistik
Ludwig-Maximilians-Universität München
Ludwigstraße 33
D-80539 München
Room 139, first floor
Phone: +49 911 58061 9595
Emilio.Dorigatti [at] stat.uni-muenchen.de