Ludwig Bothmann
About
I am a postdoctoral researcher at the Chair of Statistical Learning and Data Science in the Department of Statistics at the LMU Munich. I have a Diploma Degree (Dipl. - Stat.) in Statistics and a PhD (Dr. rer. nat.) in Statistics with focus on efficient statistical analysis of video and image data. During my PhD I worked at the Chair of Applied Statistics in Social Sciences, Economics and Business at the LMU, under the supervision of Prof. Dr. Göran Kauermann. Afterwards I worked for nearly three years as Data Scientist and Data Science Lead for an insurance company, implementing various data science use cases from first idea to production.
Since August 2020, I am part of this chair. My main research interests include fairML, uncertainty quantification, causal inference, interpretable ML and application of ML and DL in other sciences. I am leading the research group on Causal and Fair Machine Learning. I am junior member of the Munich Center for Machine Learning (MCML) and founding member of its Machine Learning Consulting Unit (MLCU).
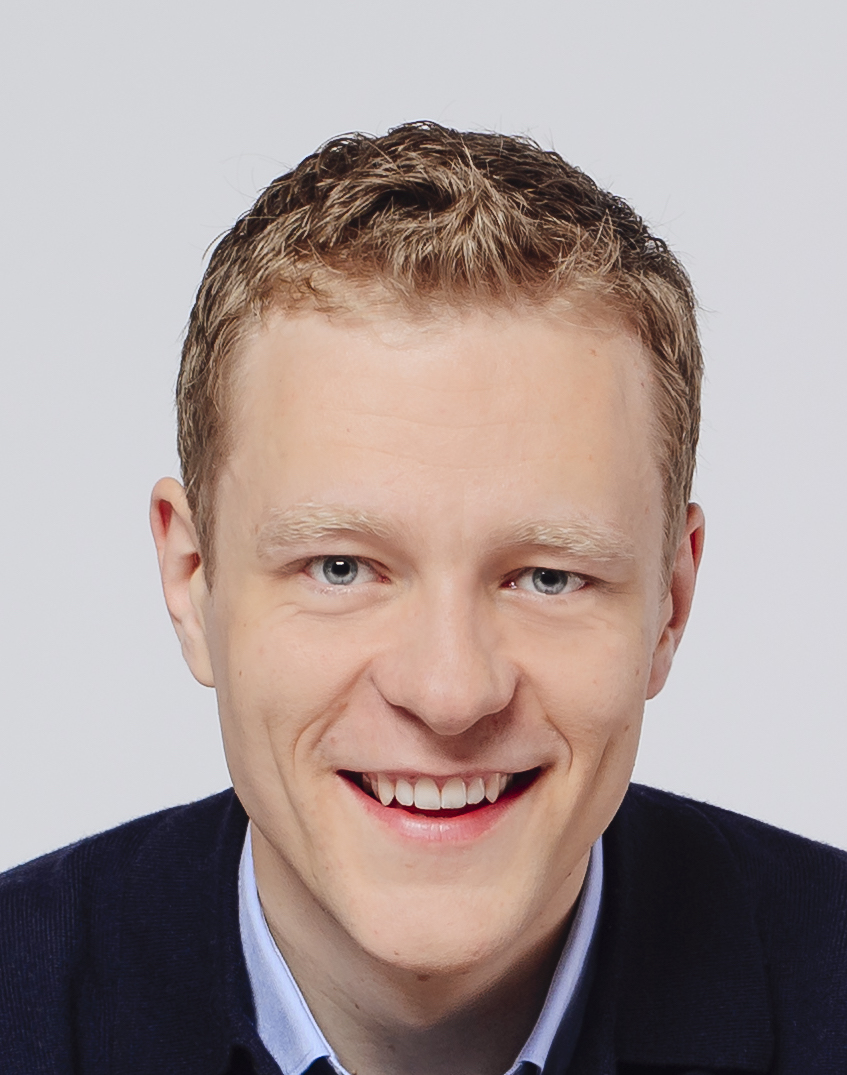
Contact
Institut für Statistik
Ludwig-Maximilians-Universität München
Ludwigstraße 33
D-80539 München
Ludwig.Bothmann [at] stat.uni-muenchen.de
You Can Find me on
Teaching
- Summer 2024:
- Winter 2023/24:
- Summer 2023:
- Winter 2022/23:
- Summer 2022:
- Winter 2021/22:
- Summer 2021:
- Winter 2020/21:
Prior teaching:
- Data Science in der Praxis: Machine Learning und Deep Learning mit Python (BA and MA Statistics):
- Lecture and Exercise: WiSe 2019/20
- R-Kurs für Nebenfachstudierende (Statistics as Minor):
- Lecture and Exercise: SoSe 2015, WiSe 2015/16, SoSe 2016, SoSe 2017, SoSe 2019
- Fortgeschrittener R-Kurs für Nebenfachstudierende (Statistics as Minor):
- Lecture and Exercise: WiSe 2017/18
- Statistik III für Nebenfachstudierende (Statistics as Minor):
- Lecture: WiSe 2014/15, WiSe 2015/16
- Exercise: WiSe 2013/14, WiSe 2014/15, WiSe 2015/16
- Schätzen und Testen I (Master Statistics)
- Exercise: WiSe 2012/13, WiSe 2013/14
- Tutorial: WiSe 2010/11, WiSe 2011/12
- Schätzen und Testen II (Master Statistics)
- Exercise: SoSe 2012, SoSe 2013, SoSe 2014, SoSe 2015, SoSe 2018
- Tutorial: SoSe 2011, SoSe 2012, SoSe 2013, SoSe 2014
- Stochastische Prozesse (Master Statistics)
- Exercise: SoSe 2013
- Versuchsplanung (Bachelor Statistics)
- Exercise: SoSe 2015
Talks
- 02.07.2024, “What Is Fairness? On the Role of Protected Attributes and Fictitious Worlds”, accepted poster at EWAF - European Workshop on Algorithmic Fairness 2024, Mainz
- 01.07.2024, “Can generative AI-based data balancing mitigate unfairness issues in Machine Learning?”, accepted poster at EWAF - European Workshop on Algorithmic Fairness 2024, Mainz
- 17.04.2024, “Causal Fair Machine Learning via Rank-Preserving Interventional Distributions”, accepted poster at EuroCIM - European Causal Inference Meeting 2024, Copenhagen, Denmark
- 01.10.2023, “Causal Fair Machine Learning via Rank-Preserving Interventional Distributions”, accepted talk at AEQUITAS Workshop on Fairness and Bias in AI at ECAI 2023, Krakow, Poland
- 07.09.2023, “Automated wildlife image classification: An active learning tool for ecological applications”, accepted talk at Camera traps, AI, and Ecology, Jena
- 24.07.2023, “Causal Fair Machine Learning via Warping Real World Data to a Fictitious, Normatively Desired World”, accepted talk at Causality for Ethics and Society, Munich
- 18.07.2023, “An active deep learning method for high out-of-sample predictive performance in image classification”, accepted poster at International Workshop on Statistical Modelling, Dortmund
- 30.03.2023, “What is fairness? Implications for fairML”, invited talk at Grenzen Künstlicher Intelligenz, Munich
- 07.09.2022, “What is fairness? Implications for fairML”, invited talk at Quality Aspects of Machine Learning - Official Statistics between Specific Quality Requirements and Methodological Innovation, Munich
- 08.02.2022, “Künstliche Intelligenz in der Strafverfolgung”, invited talk at Lecture series Cybercrime, Faculty of Law, LMU Munich
- 22.11.2021, “Kollaborative Entwicklung von Open Source Educational Resources für Machine Learning und Data Science”, invited talk at Workshop “Biometrie online lehren”, Working group (IBS-DR), Munich
- 18.02.2020, “Bilddaten: Mit Deep Learning echten Geschäftsnutzen generieren”, invited talk at German Data Science Days, LMU Munich
- 15.03.2016, “Realtime Classification of Fish in Underwater Sonar Videos”, accepted talk at DAGStat, Göttingen
- 19.03.2013, “Real Time Classification of Fish in Sonar Images (DIDSON)”, accepted talk at ECAS 2013, Castro Urdiales, Spain
References
- Felderer, B., Repke, L., Weber, W., Schweisthal, J., & Bothmann, L. (2024). Predicting the validity and reliability of survey questions. osf preprints.
link - Ronval, B., Nijssen, S., & Bothmann, L. (2024). Can generative AI-based data balancing mitigate unfairness issues in Machine Learning? Accepted at EWAF’24: European Workshop on Algorithmic Fairness.
- Dandl, S., Becker, M., Bischl, B., Casalicchio, G., & Bothmann, L. (2024). mlr3summary: Concise and interpretable summaries for machine learning models. Accepted at 2nd World Conference on eXplainable Artificial Intelligence 2024 (Demo Track).
link- Ewald, F., Bothmann, L., Wright, M., Bischl, B., Casalicchio, G., & König, G. (2024). A Guide to Feature Importance Methods for Scientific Inference. Accepted at 2nd World Conference on eXplainable Artificial Intelligence 2024.
link- Bothmann, L., & Peters, K. (2024). Fairness als Qualitätskriterium im Maschinellen Lernen – Rekonstruktion des philosophischen Konzepts und Implikationen für die Nutzung außergesetzlicher Merkmale bei qualifizierten Mietspiegeln. To appear in: AStA Wirtschafts- und Sozialstatistisches Archiv.
- Sommer, E., Wimmer, L., Papamarkou, T., Bothmann, L., Bischl, B., & Rügamer, D. (2024). Connecting the Dots: Is Mode-Connectedness the Key to Feasible Sample-Based Inference in Bayesian Neural Networks? Accepted at International Conference on Machine Learning (ICML) 2024.
link- Bothmann, L., Peters, K., & Bischl, B. (2024). What Is Fairness? On the Role of Protected Attributes and Fictitious Worlds. arXiv:2205.09622.
link- Bothmann, L., & Peters, K. (2024). Fairness von KI – ein Brückenschlag zwischen Philosophie und Maschinellem Lernen. To appear in: Rathgeber, B. & Maier, M. (Eds.), Grenzen Künstlicher Intelligenz. wbg Academic, Darmstadt.
- Hornung, R., Nalenz, M., Schneider, L., Bender, A., Bothmann, L., Bischl, B., Augustin, T., & Boulesteix, A-L. (2023) Evaluating machine learning models in non-standard settings: An overview and new findings. arXiv:2310.15108.
link- Bothmann, L., Dandl, S., & Schomaker, M. (2023) Causal Fair Machine Learning via Rank-Preserving Interventional Distributions. Proceedings of the 1st Workshop on Fairness and Bias in AI co-located with 26th European Conference on Artificial Intelligence (ECAI 2023), CEUR Workshop Proceedings, https://ceur-ws.org/Vol-3523/.
link- Dandl, S., Casalicchio, G., Bischl, B., & Bothmann, L. (2023) Interpretable Regional Descriptors: Hyperbox-Based Local Explanations. In: Koutra D, Plant C, Gomez Rodriguez M, Baralis E, Bonchi F (eds) ECML PKDD 2023: Machine Learning and Knowledge Discovery in Databases: Research Track, pp. 479–495. Springer Nature Switzerland, Cham.
link- Bothmann, L., Wimmer, L., Charrakh, O., Weber, T., Edelhoff, H., Peters, W., Nguyen, H., Benjamin, C., & Menzel, A. (2023). Automated wildlife image classification: An active learning tool for ecological applications. Ecological Informatics, 77(102231).
link | arXiv- Bothmann, L. (2022). Künstliche Intelligenz in der Strafverfolgung. In K. Peters (Ed.), Cyberkriminalität. LMU Munich.
link- Ghada, W., Casellas, E., Herbinger, J., Garcia-Benadí, A., Bothmann, L., Estrella, N., Bech, J., & Menzel, A. (2022). Stratiform and Convective Rain Classification Using Machine Learning Models and Micro Rain Radar. Remote Sensing, 14(18).
link- Bothmann, L., Strickroth, S., Casalicchio, G., Rügamer, D., Lindauer, M., Scheipl, F., & Bischl, B. (2022). Developing Open Source Educational Resources for Machine Learning and Data Science. Proceedings of the Third Teaching Machine Learning and Artificial Intelligence Workshop, PMLR 207:1-6 .
link | pdf- Matiu, M., Bothmann, L., Steinbrecher, R., & Menzel, A. (2017). Monitoring succession after a non-cleared windthrow in a Norway spruce mountain forest using webcam, satellite vegetation indices and turbulent CO 2 exchange. Agricultural and Forest Meteorology, 244–245, 72–81.
link- Bothmann, L., Menzel, A., Menze, B. H., Schunk, C., & Kauermann, G. (2017). Automated processing of webcam images for phenological classification. PLoS ONE, 12(2): e0171918.
link- Bothmann, L. (2016). Efficient statistical analysis of video and image data [PhD thesis, Ludwig-Maximilians-Universität München].
link- Bothmann, L., Windmann, M., & Kauermann, G. (2016). Realtime classification of fish in underwater sonar videos. Journal of the Royal Statistical Society: Series C (Applied Statistics), 65(4), 565–584.
link- Kalus, S., Bothmann, L., Yassouridis, C., Czisch, M., Sämann, P., & Fahrmeir, L. (2014). Statistical modeling of time-dependent fMRI activation effects. Human Brain Mapping, 36(2), 731–743.
link- Bothmann, L. (2012). Statistische Modellierung von EEG-abhängigen Stimuluseffekten in der fMRT-Analyse [Diploma Thesis].
</ol>
link - Dandl, S., Becker, M., Bischl, B., Casalicchio, G., & Bothmann, L. (2024). mlr3summary: Concise and interpretable summaries for machine learning models. Accepted at 2nd World Conference on eXplainable Artificial Intelligence 2024 (Demo Track).