Christoph Molnar
About
Since october 2017 I am a PhD student at the working group for Computational Statistics at the Ludwig-Maximilians-University Munich, doing my research on Interpretable Machine Learning.
I obtained a Bachelor's Degree (B.Sc.) and Master's Degree (M.Sc.) in Statistics from the Ludwig-Maximilians-University Munich.
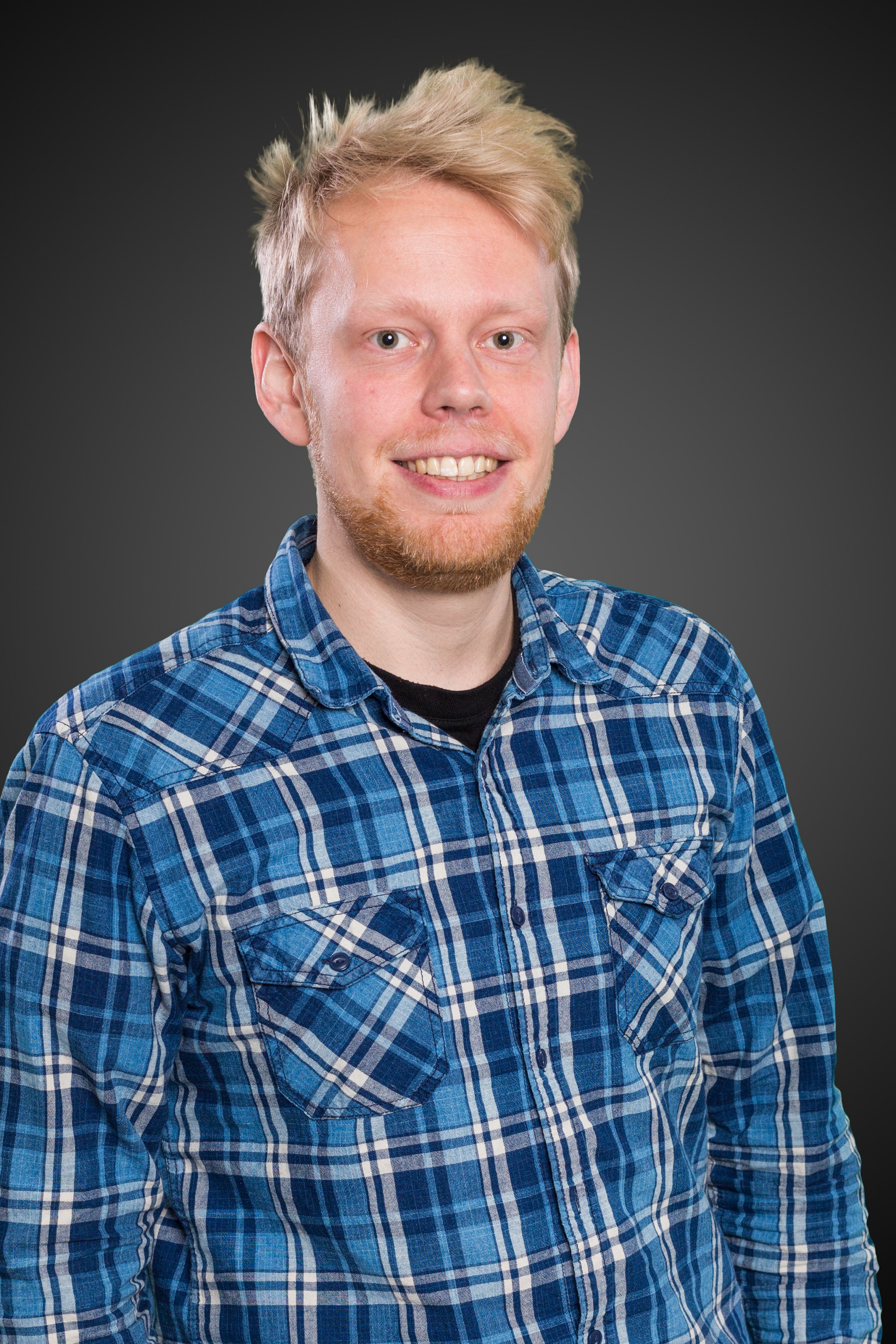
Contact
Institut für Statistik
Ludwig-Maximilians-Universität München
Ludwigstraße 33
D-80539 München
Room 139
christoph.molnar [at] stat.uni-muenchen.de
Research Interests
- Interpretable Machine learning
You Can Find me on
References
- König G, Molnar C, Bischl B, Grosse-Wentrup M (2021) Relative Feature Importance 2020 25th International Conference on Pattern Recognition (ICPR), pp. 9318–9325.
link | pdf . - Scholbeck CA, Molnar C, Heumann C, Bischl B, Casalicchio G (2020) Sampling, Intervention, Prediction, Aggregation: A Generalized Framework for Model-Agnostic Interpretations. In: In: Cellier P , In: Driessens K (eds) Machine Learning and Knowledge Discovery in Databases. ECML PKDD 2019, pp. 205–216. Springer International Publishing, Cham.
link | pdf. - Molnar C, Casalicchio G, Bischl B (2020) Quantifying Model Complexity via Functional Decomposition for Better Post-hoc Interpretability. In: In: Cellier P , In: Driessens K (eds) Machine Learning and Knowledge Discovery in Databases, pp. 193–204. Springer International Publishing, Cham.
link | pdf. - Molnar C, König G, Herbinger J, Freiesleben T, Dandl S, Scholbeck CA, Casalicchio G, Grosse-Wentrup M, Bischl B (2020) Pitfalls to Avoid when Interpreting Machine Learning Models. ICML workshop XXAI: Extending Explainable AI Beyond Deep Models and Classifiers.
link | pdf . - Molnar C, König G, Bischl B, Casalicchio G (2020) Model-agnostic Feature Importance and Effects with Dependent Features–A Conditional Subgroup Approach. arXiv preprint arXiv:2006.04628.
link | pdf . - Dandl S, Molnar C, Binder M, Bischl B (2020) Multi-Objective Counterfactual Explanations. In: In: Bäck T , In: Preuss M , In: Deutz A , In: Wang H , In: Doerr C , In: Emmerich M , In: Trautmann H (eds) Parallel Problem Solving from Nature – PPSN XVI, pp. 448–469. Springer International Publishing, Cham.
link | video. - Molnar C, Casalicchio G, Bischl B (2020) Interpretable Machine Learning–A Brief History, State-of-the-Art and Challenges. arXiv preprint arXiv:2010.09337.
link | pdf . - Casalicchio G, Molnar C, Bischl B (2019) Visualizing the Feature Importance for Black Box Models. In: In: Berlingerio M , In: Bonchi F , In: Gärtner T , In: Hurley N , In: Ifrim G (eds) Machine Learning and Knowledge Discovery in Databases. ECML PKDD 2018, pp. 655–670. Springer International Publishing, Cham.
link | pdf . - Molnar C (2019) Interpretable Machine Learning.
link. - Molnar C, Casalicchio G, Bischl B (2018) iml: An R package for Interpretable Machine Learning. The Journal of Open Source Software 3, 786.
link | pdf .