Mina Rezaei
About
I am a Postdoc at the Department of Statistics at the Ludwig-Maximilians-University Munich (LMU) at the chair of Statistical Learning and Data Science. Previously, I have been a machine learning/deep learning researcher at Hasso-Plattner Institute (HPI), Potsdam University under the supervision of Prof. Dr. Christoph Meinel. I did my M.Sc. degree in Artificial Intelligence at the Department of Computer Science, Shiraz University and Bachelor's degree in Computer Science, Software Engineering.
My research interests include both technical and theoretical skills of machine learning and health care applications.
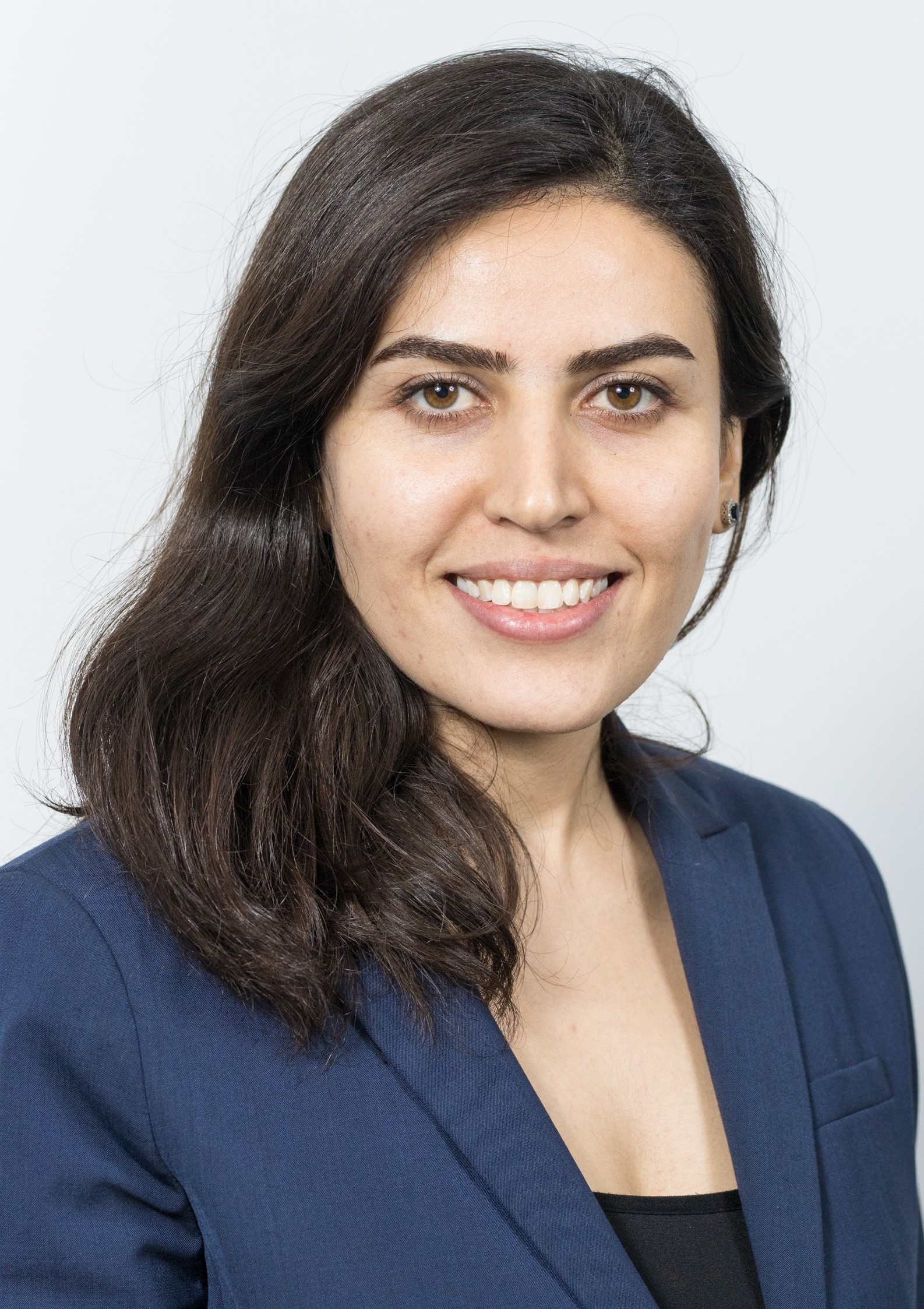
Contact
Institut für Statistik
Ludwig-Maximilians-Universität München
Ludwigstraße 33
D-80539 München
Mina.Rezaei [at] stat.uni-muenchen.de
Teaching
- Summer 2023:
- Winter 2022/23:
- Summer 2022:
- Winter 2021/22:
- Summer 2021:
- Winter 2020/21:
Thesis
I offer different master thesis topics such as deep un/self-supervised learning, deep metric learning, deep learning application in health care or in computer vision. If you are interested, please send me your topic of interest, your CV, and your current transcript.
Research Interests
- Deep un/semi/self-supervised learning
- Probabilistic deep learning
- Deep learning application in health care
- Deep learning application in computer vision and multimodal dataset
You Can Find me on
Recent Publications
- Rezaei M, Soleymani F, Bischl B, Azizi S (2023) Deep bregman divergence for contrastive learning of visual representations. Computer Vision and Image Understanding.
- Saggau D, Rezaei M, Bisch B, Chalkidis I (2023) Efficient Document Embeddings via Self-Contrastive Bregman Divergence Learning. The 61st Annual Meeting of the Association for Computational Linguistics.
- Rezaei M, Vahidi A, Bischl B, Wang M, Elze T, Eslami M (2023) Self-supervised Learning and Self-labeling Framework for Retina Glaucoma Detection. Investigative Ophthalmology & Visual Science 64, 352–352.
- Turkoglu MO, Becker A, Gündüz HA, Rezaei M, Bischl B, Daudt RC, D’Aronco S, Wegner J, Schindler K (2022) Film-ensemble: Probabilistic deep learning via feature-wise linear modulation. Advances in Neural Information Processing Systems 35, 22229–22242.
- Rezaei M, Dorigatti E, Rügamer D, Bischl B (2022) Joint Debiased Representation Learning and Imbalanced Data Clustering 2022 IEEE International Conference on Data Mining Workshops (ICDMW), pp. 55–62. IEEE.
- Rezaei M, Näppi J, Bischl B, Yoshida H (2022) Deep mutual GANs: representation learning from multiple experts Medical Imaging Analysis: Imaging Informatics for Healthcare, Research, and Applications, pp. 191–197.
- Rezaei M, Näppi JJ, Bischl B, Yoshida H (2022) Bayesian uncertainty estimation for detection of long-tail and unseen conditions in abdominal images Medical Imaging Analysis: Computer-Aided Diagnosis, pp. 270–276.
- Rezaei M, Uemura T, Näppi J, Yoshida H, Lippert C, Meinel C (2020) Generative synthetic adversarial network for internal bias correction and handling class imbalance problem in medical image diagnosis Medical Imaging 2020: Computer-Aided Diagnosis, p. 113140E. International Society for Optics and Photonics.
- Rezaei M, Yang H, Meinel C (2019) Learning imbalanced semantic segmentation through cross-domain relations of multi-agent generative adversarial networks Medical Imaging 2019: Computer-Aided Diagnosis, p. 1095027. International Society for Optics and Photonics.
- Rezaei M, Yang H, Harmuth K, Meinel C (2019) Conditional generative adversarial refinement networks for unbalanced medical image semantic segmentation 2019 IEEE Winter Conference on Applications of Computer Vision (WACV), pp. 1836–1845. IEEE.
- Rezaei M, Yang H, Meinel C (2019) Recurrent generative adversarial network for learning imbalanced medical image semantic segmentation. Multimedia Tools and Applications, 1–20.
- Rezaei M, Yang H, Meinel C (2019) voxel-gan: Adversarial framework for learning imbalanced brain tumor segmentation. BrainLes 2018. Springer LNCS 11384, 321–333.
- Rezaei M, Yang H, Meinel C (2018) Instance tumor segmentation using multitask convolutional neural network 2018 International Joint Conference on Neural Networks (IJCNN), pp. 1–8. IEEE.
- Bakas S, Reyes M, Jakab A, Bauer S, Rempfler M, Crimi A, Shinohara RT, Berger C, Ha SM, Rozycki M, others (2018) Identifying the best machine learning algorithms for brain tumor segmentation, progression assessment, and overall survival prediction in the BRATS challenge. arXiv preprint arXiv:1811.02629.
- Rezaei M, Harmuth K, Gierke W, Kellermeier T, Fischer M, Yang H, Meinel C (2017) A conditional adversarial network for semantic segmentation of brain tumor International MICCAI Brainlesion Workshop, pp. 241–252. Springer.