Mina Rezaei
About
I am a junior group leader and university lecturer at the Chair of Statistical Learning and Data Science, Department of Statistics, LMU Munich. Currently, I serve as the teaching coordinator for the Munich Center for Machine Learning (MCML) and lead the "Methods Beyond Supervised Learning" focus group at LMU Munich's Statistics Department. Before joining LMU, I was a research fellow at Harvard Medical School and Mass General Hospital in Boston, working with Hiroyuki Yoshida's group. During that time, I focused on probabilistic deep learning and robust representation learning for clinical data analysis. I earned my Ph.D. from the Hasso Plattner Institute (HPI) at Potsdam University, where I conducted research on robust deep learning models for medical image analysis under the supervision of Prof. Christoph Meinel. I also hold an M.Sc. in Artificial Intelligence from the Department of Computer Science, Shiraz University, and a Bachelor's degree in Computer Science, Software Engineering.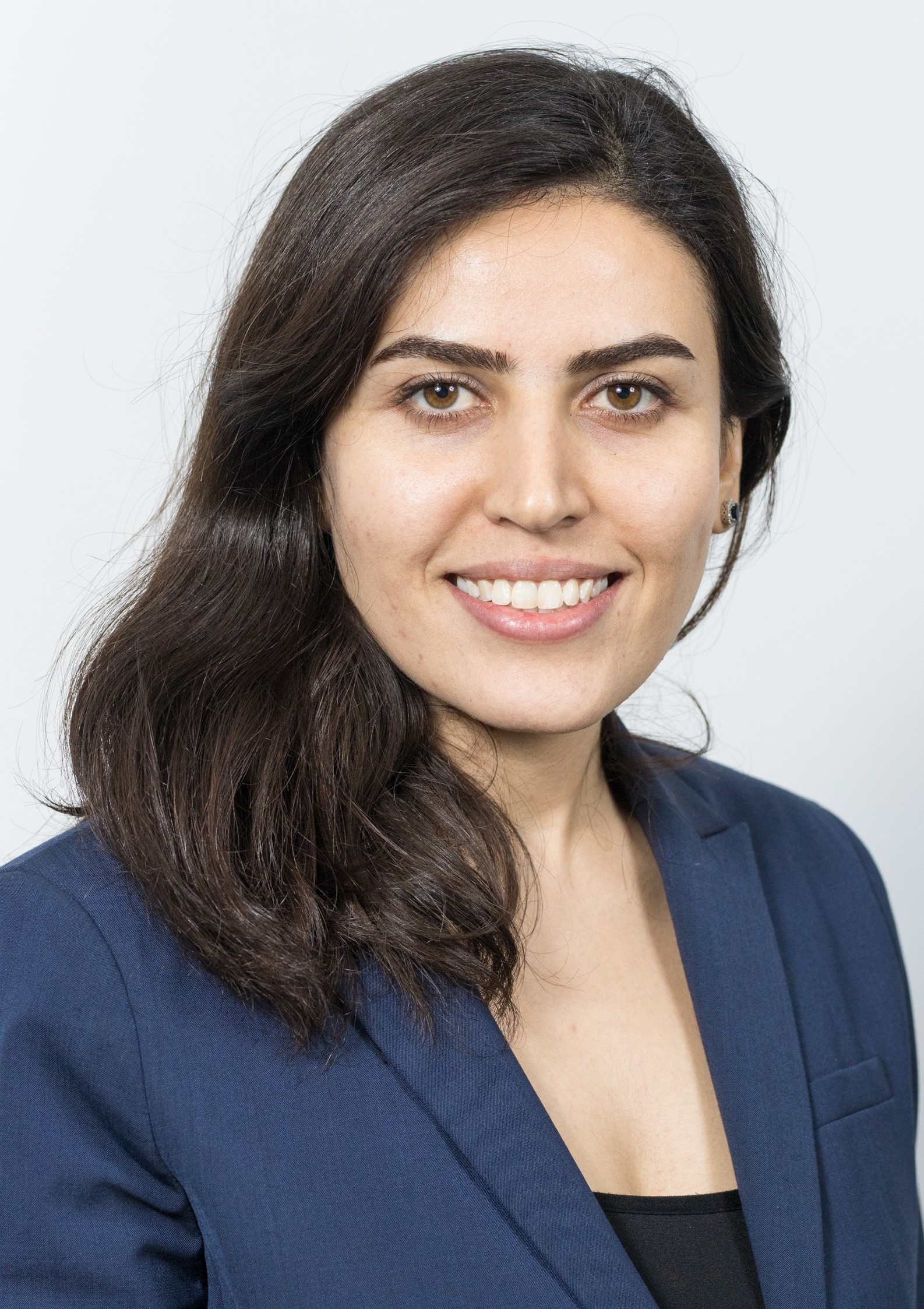
Contact
Ludwig-Maximilians-Universität München
Institut für Statistik
Ludwigstr. 33,80539 Munich, Germany
Mina.Rezaei [at] stat.uni-muenchen.de
Research Interests
My research interests include both technical and theoretical skills of machine learning with a focus on its transformative applications within the realm of computer vision, natural language processing (NLP), and healthcare:
-
Learning with minimal supervision (semi-supervised learning, positive-unlabeled learning, self-supervised learning)
-
Probabilistic machine learning (scoring rule, Bayesian neural networks, uncertainty estimation)
-
Deep metric learning (geometry learning, contrastive learning, ranking)
-
Generative models (GANs, diffusion, flow models, consistency models)
Teaching
- Summer 2025:
- Winter 2024/25:
- I was on parental- leave in winter 2023/24 and summer 2024.
- Summer 2023:
- Winter 2022/23:
- Summer 2022:
- Winter 2021/22:
- Summer 2021:
- Winter 2020/21:
Thesis
I offer different master thesis topics such as deep un/self-supervised learning, deep metric learning, deep learning application in health care or in computer vision. If you are interested, please send me your topic of interest, your CV, and your current transcript.
You Can Find me on
Selected Publications
- Li Y, Rügamer D, Bischl B, Rezaei M (2025) Calibrating LLMs with Information-Theoretic Evidential Deep Learning The Thirteenth International Conference on Learning Representations (ICLR),
link. - Li* Y, Zhang* Y, Kawaguchi K, Khakzar A, Bischl B, Rezaei M (2024) A Dual-Perspective Approach to Evaluating Feature Attribution Methods. Transactions on Machine Learning Research (TMLR).
link. - Zhang* Y, Li* Y, Wang X, Shen Q, Plank B, Bischl B, Rezaei M, Kawaguchi K (2024) FinerCut: Finer-grained Interpretable Layer Pruning for Large Language Models. Compression Workshop at NeurIPS 2024.
link. - Vahidi A, Schosser S, Wimmer L, Li Y, Bischl B, Hüllermeier E, Rezaei M (2024) Probabilistic Self-supervised Representation Learning via Scoring Rules Minimization The Twelfth International Conference on Learning Representations,
- Nam J, Chalkidis I, Rezaei M (2024) Hyperbolic Contrastive Learning for Document Representations–A Multi-View Approach with Paragraph-level Similarities ECAI 2024, pp. 1656–1663. IOS Press.
- Vahidi A, Wimmer L, Gündüz HA, Bischl B, Hüllermeier E, Rezaei M (2024) Diversified ensemble of independent sub-networks for robust self-supervised representation learning Joint European Conference on Machine Learning and Knowledge Discovery in Databases, pp. 38–55. Springer.
- Stermann F, Chalkidis I, Vahidi A, Bischl B, Rezaei M (2024) Attention-Driven Dropout: A Simple Method to Improve Self-supervised Contrastive Sentence Embeddings Joint European Conference on Machine Learning and Knowledge Discovery in Databases, pp. 89–106. Springer.
- Erick FX, Rezaei M, Müller JP, Kainz B (2024) Uncertainty-Aware Vision Transformers for Medical Image Analysis International Workshop on Uncertainty for Safe Utilization of Machine Learning in Medical Imaging, pp. 171–180. Springer.
- Gündüz HA, Mreches R, Moosbauer J, Robertson G, To X-Y, Franzosa EA, Huttenhower C, Rezaei M, McHardy AC, Bischl B, others (2024) Optimized model architectures for deep learning on genomic data. Communications Biology 7, 516.
- Olayo-Alarcon R, Amstalden MK, Zannoni A, Bajramovic M, Sharma CM, Brochado AR, Rezaei M, Müller CL (2024) Pre-trained molecular representations enable antimicrobial discovery. bioRxiv, 2024–03.
- Gündüz HA, Binder M, To X-Y, Mreches R, Bischl B, McHardy AC, Münch PC, Rezaei M (2023) A self-supervised deep learning method for data-efficient training in genomics. Communications Biology 6, 928.
- Gündüz HA, Giri S, Binder M, Bischl B, Rezaei M (2023) Uncertainty Quantification for Deep Learning Models Predicting the Regulatory Activity of DNA Sequences 2023 International Conference on Machine Learning and Applications (ICMLA), pp. 566–573. IEEE.
- Scheppach A, Gündüz HA, Dorigatti E, Münch PC, McHardy AC, Bischl B, Rezaei M, Binder M (2023) Neural Architecture Search for Genomic Sequence Data 2023 IEEE Conference on Computational Intelligence in Bioinformatics and Computational Biology (CIBCB), pp. 1–10. IEEE.
- Rezaei M, Näppi JJ, Bischl B, Yoshida H (2023) Bayesian uncertainty estimation for detection of long-tailed and unseen conditions in medical images. Journal of Medical Imaging 10, 054501–054501.
- Rezaei M, Soleymani F, Bischl B, Azizi S (2023) Deep bregman divergence for contrastive learning of visual representations. Computer Vision and Image Understanding.
- Saggau D, Rezaei M, Bisch B, Chalkidis I (2023) Efficient Document Embeddings via Self-Contrastive Bregman Divergence Learning. The 61st Annual Meeting of the Association for Computational Linguistics.
- Rezaei M, Vahidi A, Bischl B, Wang M, Elze T, Eslami M (2023) Self-supervised Learning and Self-labeling Framework for Retina Glaucoma Detection. Investigative Ophthalmology & Visual Science 64, 352–352.
- Turkoglu MO, Becker A, Gündüz HA, Rezaei M, Bischl B, Daudt RC, D’Aronco S, Wegner J, Schindler K (2022) Film-ensemble: Probabilistic deep learning via feature-wise linear modulation. Advances in Neural Information Processing Systems 35, 22229–22242.
- Rezaei M, Dorigatti E, Rügamer D, Bischl B (2022) Joint Debiased Representation Learning and Imbalanced Data Clustering 2022 IEEE International Conference on Data Mining Workshops (ICDMW), pp. 55–62. IEEE.
- Rezaei M, Näppi J, Bischl B, Yoshida H (2022) Deep mutual GANs: representation learning from multiple experts Medical Imaging Analysis: Imaging Informatics for Healthcare, Research, and Applications, pp. 191–197.
- Rezaei M, Näppi JJ, Bischl B, Yoshida H (2022) Bayesian uncertainty estimation for detection of long-tail and unseen conditions in abdominal images Medical Imaging Analysis: Computer-Aided Diagnosis, pp. 270–276.
- Rezaei M, Uemura T, Näppi J, Yoshida H, Lippert C, Meinel C (2020) Generative synthetic adversarial network for internal bias correction and handling class imbalance problem in medical image diagnosis Medical Imaging 2020: Computer-Aided Diagnosis, p. 113140E. International Society for Optics and Photonics.
- Rezaei M, Yang H, Meinel C (2019) Learning imbalanced semantic segmentation through cross-domain relations of multi-agent generative adversarial networks Medical Imaging 2019: Computer-Aided Diagnosis, p. 1095027. International Society for Optics and Photonics.
- Rezaei M, Yang H, Harmuth K, Meinel C (2019) Conditional generative adversarial refinement networks for unbalanced medical image semantic segmentation 2019 IEEE Winter Conference on Applications of Computer Vision (WACV), pp. 1836–1845. IEEE.
- Rezaei M, Yang H, Meinel C (2019) Recurrent generative adversarial network for learning imbalanced medical image semantic segmentation. Multimedia Tools and Applications, 1–20.
- Rezaei M, Yang H, Meinel C (2019) voxel-gan: Adversarial framework for learning imbalanced brain tumor segmentation. BrainLes 2018. Springer LNCS 11384, 321–333.
- Rezaei M, Yang H, Meinel C (2018) Instance tumor segmentation using multitask convolutional neural network 2018 International Joint Conference on Neural Networks (IJCNN), pp. 1–8. IEEE.
- Bakas S, Reyes M, Jakab A, Bauer S, Rempfler M, Crimi A, Shinohara RT, Berger C, Ha SM, Rozycki M, others (2018) Identifying the best machine learning algorithms for brain tumor segmentation, progression assessment, and overall survival prediction in the BRATS challenge. arXiv preprint arXiv:1811.02629.
- Rezaei M, Harmuth K, Gierke W, Kellermeier T, Fischer M, Yang H, Meinel C (2017) A conditional adversarial network for semantic segmentation of brain tumor International MICCAI Brainlesion Workshop, pp. 241–252. Springer.