Prof. Dr. Matthias Feurer
About
I am a Thomas Bayes Fellow and interim professor at the Chair of Statistical Learning and Data Science funded by the Munich Center for Machine Learning.
With my research, I aim to simplify the usage of machine learning by researching methods and developing tools that allow the usage of machine learning by domain scientists and also make machine learning more efficient for expert users. My focus is on Automated Machine Learning (AutoML) which encompasses methods for hyperparameter optimization, meta-learning, and model selection. However, machine learning is not solely about predictive performance but also about additional objectives such as model interpretability, deployability, or fairness. For this, I want to move beyond performance-driven machine learning by applying multi-objective AutoML.
Furthermore, I am developing and contributing to multiple open-source projects in the realm of AutoML (see below) and am a co-founder of the Open Machine Learning Foundation that supports the development of OpenML.org.
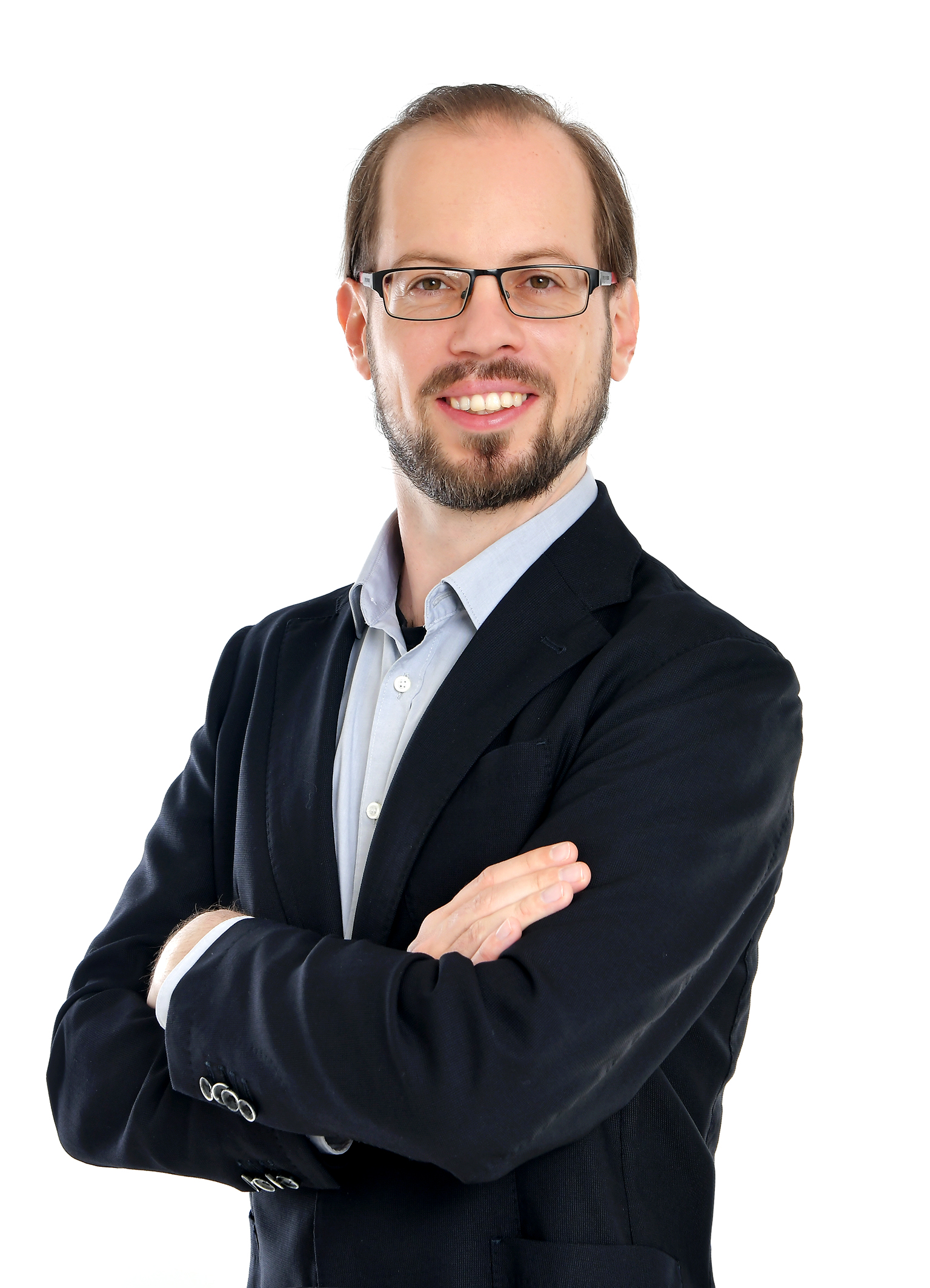
Short Bio
Since April 2023, Matthias Feurer is a Thomas Bayes Fellow and interim professor, funded by the Munich Center of Machine Learning (MCML). He is a member of the Chair of Statistical Learning and Data Science (Department of Statistics, LMU Munich), which is headed by Prof. Dr. Bernd Bischl. Previously, he was a PostDoc at the machine learning lab at the Albert-Ludwigs-Universität Freiburg, where he also finished his PhD under the supervision of Prof. Dr. Frank Hutter on the topic of Automated Machine Learning. Before that, he studied Computer Science at the University of Freiburg (M.Sc.) and Computer Science and Media at the Media University Stuttgart (B.Sc.).
Contact
Institut für Statistik
Ludwig-Maximilians-Universität München
Room 140
Ludwigstraße 33
D-80539 München
matthias.feurer [at] stat.uni-muenchen.de
Phone: +49 89 2180 3847
Teaching
- Winter Term 2024/2025
- Summer Term 2024
- Winter Term 2023/2024
- Summer Term 2023
Thesis supervision
Please see the AutoML thesis topics on Moodle.
Research Interests
My main research interest lies in the field of Automated Machine Learning (AutoML):
- AutoML Systems
- Hyperparameter Optimization
- Bayesian Optimization
- Meta-Learning
Other topics that interest me:
- Empirical Machine Learning
- Open Source Software
You Can Find me on
Projects
- auto-sklearn: An automated machine learning solution that simplifies solving supervised classification and regression problems.
- OpenML-Python: Python API to interact with OpenML.org
- SMAC3: a reimplementation of the SMAC method in Python
- HPOBench: Hyperparameter Optimization benchmarks library
Discontinued
- HPOlib 1.5: 2nd iteration of the Hyperparameter Optimization library, discontinued
- HPOlib: Hyperparameter Optimization library, discontinued
Other
- I gave an invited talk at the AutoML workshop describing our work on Auto-sklearn 2.0 which can be found on Slideslive.
- Together with Katharina I gave an introduction to Auto-sklearn at the EuroPython 2021 which can be found on Youtube.
- We placed 1st in the warmstarting-friendly leaderboard of the BBO NeurIPS challenge.
- We wrote a blog post about meta-learning the arguments to Auto-sklearn, check it out here.
- Read our blog post on winning the 2nd AutoML challenge.
- Read our winning blog post of the kdnuggets blog contest on AutoML.
- Check out this interview with Aaron, Frank and me about the winning the AutoML challenge.
- Our work on Auto-sklearn got covered in the Computer Vision News.
Publications
- Nagler T, Schneider L, Bischl B, Feurer M (2025) Reshuffling Resampling Splits Can Improve Generalization of Hyperparameter Optimization Advances in Neural Information Processing Systems, pp. 40486–40533.
Link | PDF | arXiv | Code | Conference Video | AutoML Seminar Video. - Koshil M, Nagler T, Feurer M, Eggensperger K (2024) Towards Localization via Data Embedding for TabPFN NeurIPS 2024 Third Table Representation Learning Workshop,
Link | PDF. - Bergman E, Feurer M, Bahram A, Balef AR, Purucker L, Segel S, Lindauer M, Hutter F, Eggensperger K (2024) AMLTK: A Modular AutoML Toolkit in Python. Journal of Open Source Software 9, 6367.
Link | PDF | Code | Video. - Lindauer M, Karl F, Klier A, Moosbauer J, Tornede A, Müller AC, Hutter F, Feurer M, Bischl B (2024) Position: A Call to Action for a Human-Centered AutoML Paradigm Proceedings of the 41st International Conference on Machine Learning, pp. 30566–30584.
Link | PDF | arXiv | Video | Blog. - Herrmann M, Lange FJD, Eggensperger K, Casalicchio G, Wever M, Feurer M, Rügamer D, Hüllermeier E, Boulesteix A-L, Bischl B (2024) Position: Why We Must Rethink Empirical Research in Machine Learning Proceedings of the 41st International Conference on Machine Learning, pp. 18228–18247.
Link | PDF | arXiv. - Kohli R, Feurer M, Bischl B, Eggensperger K, Hutter F (2024) Towards Quantifying the Effect of Datasets for Benchmarking: A Look at Tabular Machine Learning Data-centric Machine Learning (DMLR) workshop at the International Conference on Learning Representations (ICLR),
Link | PDF | Code. - Rundel D, Kobialka J, von Crailsheim C, Feurer M, Nagler T, Rügamer D (2024) Interpretable Machine Learning for TabPFN Explainable Artificial Intelligence, pp. 465–476.
Link | PDF | arXiv | Code. - Weerts H, Pfisterer F, Feurer M, Eggensperger K, Bergman E, Awad N, Vanschoren J, Pechenizkiy M, Bischl B, Hutter F (2024) Can Fairness be Automated? Guidelines and Opportunities for Fairness-aware AutoML. Journal of Artificial Intelligence Research 79, 639–677.
Link | PDF | arXiv | Blog. - Müller S, Feurer M, Hollmann N, Hutter F (2023) PFNs4BO: In-Context Learning for Bayesian Optimization Proceedings of the 40th International Conference on Machine Learning, pp. 25444–25470.
Link | PDF | arXiv | Code | Video | Blog. - Feurer M, Eggensperger K, Bergman E, Pfisterer F, Bischl B, Hutter F (2023) Mind the Gap: Measuring Generalization Performance Across Multiple Objectives Advances in Intelligent Data Analysis XXI. IDA 2023., pp. 130–142.
Link | PDF | arXiv | Code. - Fischer S, Harutyunyan L, Feurer M, Bischl B (2023) OpenML-CTR23 – A curated tabular regression benchmarking suite AutoML Conference 2023 (Workshop),
Link | PDF | Code | Video | Teaser. - Müller S, Arango SP, Feurer M, Grabocka J, Hutter F (2022) Bayesian Optimization with a Neural Network Meta-learned on Synthetic Data Only 6th NeurIPS Workshop on Meta-Learning,
Link | PDF | Video. - Feurer M, Eggensperger K, Falkner S, Lindauer M, Hutter F (2022) Auto-Sklearn 2.0: Hands-free AutoML via Meta-Learning. Journal of Machine Learning Research 23, 1–61.
Link | PDF | arXiv | Code | Blog. - Lindauer M, Eggensperger K, Feurer M, Biedenkapp A, Deng D, Benjamins C, Ruhkopf T, Sass R, Hutter F (2022) SMAC3: A Versatile Bayesian Optimization Package for Hyperparameter Optimization. Journal of Machine Learning Research 23, 1–9.
Link | PDF | arXiv | Code | Video | https://www.youtube.com/watch?v=Pz3FoPr-Z5w. - Feurer M (2022) Robust and Efficient Automated Machine Learning: Systems, Infrastructure and Advances in Hyperparameter Optimization.
Link | PDF. - Feurer M, Letham B, Hutter F, Bakshy E (2022) Practical Transfer Learning for Bayesian Optimization. arXiv:1802.02219v4 [cs.LG].
arXiv | PDF | Code. - Bischl B, Casalicchio G, Feurer M, Gijsbers P, Hutter F, Lang M, Mantovani RG, Rijn JN van, Vanschoren J (2021) OpenML Benchmarking Suites Proceedings of the Neural Information Processing Systems Track on Datasets and Benchmarks,
Link | PDF | arXiv | Video. - Eggensperger K, Müller P, Mallik N, Feurer M, Sass R, Klein A, Awad N, Lindauer M, Hutter F (2021) HPOBench: A Collection of Reproducible Multi-Fidelity Benchmark Problems for HPO Proceedings of the Neural Information Processing Systems Track on Datasets and Benchmarks,
Link | PDF | arXiv | Code | Video. - Feurer M, Rijn JN van, Kadra A, Gijsbers P, Mallik N, Ravi S, Müller A, Vanschoren J, Hutter F (2021) OpenML-Python: an extensible Python API for OpenML. Journal of Machine Learning Research 22, 1–5.
Link | PDF | arXiv | Code. - Awad N, Shala G, Deng D, Mallik N, Feurer M, Eggensperger K, Biedenkapp A, Vermetten D, Wang H, Doerr C, Lindauer M, Hutter F (2020) Squirrel: A Switching Hyperparameter Optimizer Description of the entry by AutoML.org & IOHprofiler to the NeurIPS 2020 BBO challenge. arXiv:2012.08180 [cs.LG].
arXiv | PDF | Code. - Lindauer M, Eggensperger K, Feurer M, Biedenkapp A, Marben J, Müller P, Hutter F (2019) BOAH: A Tool Suite for Multi-Fidelity Bayesian Optimization & Analysis of Hyperparameters. arXiv:1908.06756 [cs.LG].
arXiv | PDF | Code. - Feurer M, Hutter F (2019) Hyperparameter Optimization AutoML: Methods, Sytems, Challenges, pp. 3–38. Springer.
Link | PDF. - Feurer M, Klein A, Eggensperger K, Springenberg JT, Blum M, Hutter F (2019) Auto-sklearn: Efficient and Robust Automated Machine Learning AutoML: Methods, Sytems, Challenges, pp. 113–134. Springer.
Link | PDF | Code. - Mendoza H, Klein A, Feurer M, Springenberg JT, Urban M, Burkart M, Dippel M, Lindauer M, Hutter F (2019) Towards Automatically-Tuned Deep Neural Networks AutoML: Methods, Sytems, Challenges, pp. 135–149. Springer.
Link | PDF | Code. - Lindauer M, Feurer M, Eggensperger K, Biedenkapp A, Hutter F (2019) Towards Assessing the Impact of Bayesian Optimization’s Own Hyperparameters IJCAI 2019 Data Science Meets Optimisation Workshop,
arXiv | PDF. - Feurer M, Letham B, Bakshy E (2018) Scalable Meta-Learning for Bayesian Optimization using Ranking-Weighted Gaussian Process Ensembles ICML 2018 AutoML Workshop,
Link | PDF. - Feurer M, Eggensperger K, Falkner S, Lindauer M, Hutter F (2018) Practical Automated Machine Learning for the AutoML Challenge 2018 ICML 2018 AutoML Workshop,
Link | PDF | Blog. - Feurer M, Hutter F (2018) Towards Further Automation in AutoML ICML 2018 AutoML Workshop,
Link | PDF. - Mendoza H, Klein A, Feurer M, Springenberg JT, Hutter F (2016) Towards Automatically-Tuned Neural Networks ICML 2016 AutoML Workshop,
- Feurer M, Klein A, Eggensperger K, Springenberg JT, Blum M, Hutter F (2015) Efficient and Robust Automated Machine Learning Advances in Neural Information Processing Systems, pp. 2962–2970.
Link | PDF | Code. - Feurer M, Klein A, Eggensperger K, Springenberg JT, Blum M, Hutter F (2015) Methods for Improving Bayesian Optimization for AutoML ICML 2015 AutoML Workshop,
- Vanschoren J, Rijn JN van, Bischl B, Casalicchio G, Lang M, Feurer M (2015) OpenML: a Networked Science Platform for Machine Learning (Abstract) ICML Workshop on Machine Learning Open Source Software,
- Feurer M, Springenberg JT, Hutter F (2015) Initializing Bayesian Hyperparameter Optimization via Meta-Learning Proceedings of the AAAI Conference on Artificial Intelligence,
Link | PDF. - Feurer M, Springenberg JT, Hutter F (2014) Using Meta-Learning to Initialize Bayesian Optimization of Hyperparameters ECAI workshop on Metalearning and Algorithm Selection (MetaSel),
Link | PDF. - Eggensperger K, Feurer M, Hutter F, Bergstra J, Snoek J, Hoos HH, Leyton-Brown K (2013) Towards an Empirical Foundation for Assessing Bayesian Optimization of Hyperparameters NeurIPS workshop on Bayesian Optimization in Theory and Practice,
Link | Code.