Dr. Susanne Dandl
About
I started as a PhD student at the Chair of Statistical Learning and Data Science of the Department of Statistics at the LMU in October 2019 focussing on causality concepts in machine learning, namely estimating heterogeneous treatment effects with machine learning and interpreting machine learning models post-hoc using counterfactual explanations and semi-factual explanations.
Since December 2023, I am supporting the chair as a Postdoc, still focusing on interpretable machine learning as well as research software engineering.
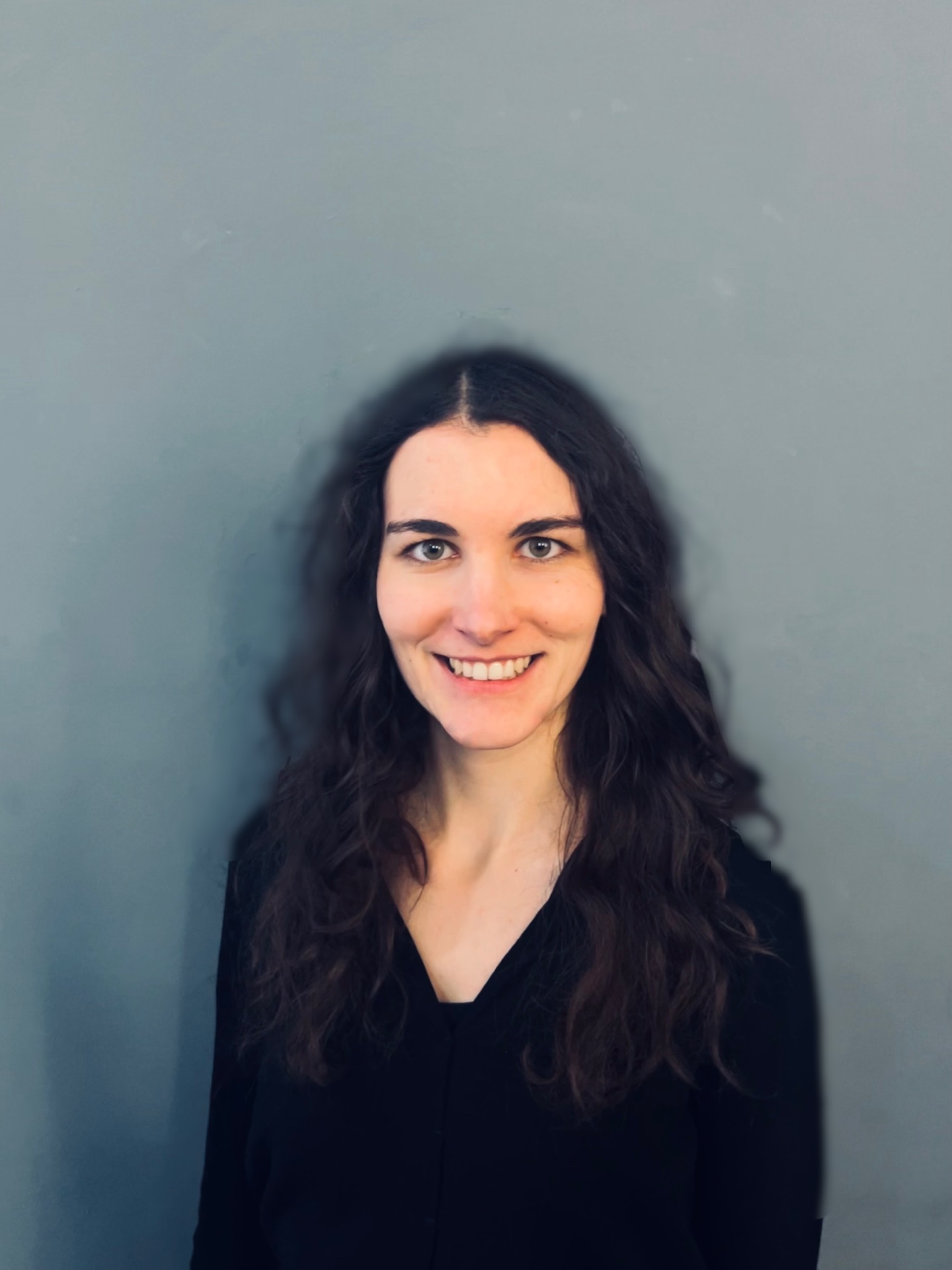
Contact
Institut für Statistik
Ludwig-Maximilians-Universität München
Ludwigstraße 33
D-80539 München
Susanne.Dandl [at] stat.uni-muenchen.de
Research Interests
- Interpreting machine learning models with counterfactual explanations
- Estimating heterogeneous treatment effects with machine learning
- Research software engineering
You can find me on
References
- Dandl S, Becker M, Bischl B, Casalicchio G, Bothmann L (2024) mlr3summary: Concise and interpretable summaries for machine learning models. Proceedings of the Demo Track of the 2nd World Conference on eXplainable Artificial Intelligence.
link|pdf. - Dandl S, Blesch K, Freiesleben T, König G, Kapar J, Bischl B, Wright M (2024) CountARFactuals – Generating plausible model-agnostic counterfactual explanations with adversarial random forests 2nd World Conference on eXplainable Artificial Intelligence,
link|pdf. - Dandl S, Haslinger C, Hothorn T, Seibold H, Sverdrup E, Wager S, Zeileis A (2024) What Makes Forest-Based Heterogeneous Treatment Effect Estimators Work? The Annals of Applied Statistics 18, 506–528.
link. - Dandl S, Bender A, Hothorn T (2024) Heterogeneous treatment effect estimation for observational data using model-based forests. Statistical Methods in Medical Research 33, 392–413.
link. - Herbinger J, Dandl S, Ewald FK, Loibl S, Casalicchio G (2024) Leveraging Model-Based Trees as Interpretable Surrogate Models for Model Distillation. In: In: Nowaczyk Set al. (ed) Artificial Intelligence. ECAI 2023 International Workshops, pp. 232–249. Springer Nature Switzerland, Cham.
link | pdf. - Dandl S, Casalicchio G, Bischl B, Bothmann L (2023) Interpretable Regional Descriptors: Hyperbox-Based Local Explanations. In: In: Koutra D , In: Plant C , In: Gomez Rodriguez M , In: Baralis E , In: Bonchi F (eds) Machine Learning and Knowledge Discovery in Databases: Research Track (ECML PKDD 2023), pp. 479–495. Springer Nature Switzerland, Cham.
link|arXiv. - Bothmann L, Dandl S, Schomaker M (2023) Causal Fair Machine Learning via Rank-Preserving Interventional Distributions. arXiv:2307.12797 [cs, stat].
link. - Dandl S, Hofheinz A, Binder M, Bischl B, Casalicchio G (2023) counterfactuals: An R Package for Counterfactual Explanation Methods. arXiv preprint arXiv:2304.06569.
link|pdf. - Dandl S, Pfisterer F, Bischl B (2022) Multi-Objective Counterfactual Fairness Proceedings of the Genetic and Evolutionary Computation Conference Companion, pp. 328–331. Association for Computing Machinery, New York, NY, USA.
link. - Molnar C, König G, Herbinger J, Freiesleben T, Dandl S, Scholbeck CA, Casalicchio G, Grosse-Wentrup M, Bischl B (2022) General Pitfalls of Model-Agnostic Interpretation Methods for Machine Learning Models xxAI - Beyond Explainable AI, pp. 39–68. Springer International Publishing.
link | pdf. - Pfisterer F, Kern C, Dandl S, Sun M, Kim MP, Bischl B (2021) mcboost: Multi-Calibration Boosting for R. Journal of Open Source Software 6, 3453.
link. - Dandl S, Molnar C, Binder M, Bischl B (2020) Multi-Objective Counterfactual Explanations. In: In: Bäck T , In: Preuss M , In: Deutz A , In: Wang H , In: Doerr C , In: Emmerich M , In: Trautmann H (eds) Parallel Problem Solving from Nature – PPSN XVI, pp. 448–469. Springer International Publishing, Cham.
link | video.